Feedback
Feedbacks help you review model generated response, making sure they are meeting your business's expectations.
In order to improve your Gen AI application, it's crucial to qualify and review the outputs generated by models.
Giving feedback can either validate the good results of a model or outline problematic generations, to learn more about all types of feedback, see Feedback types.
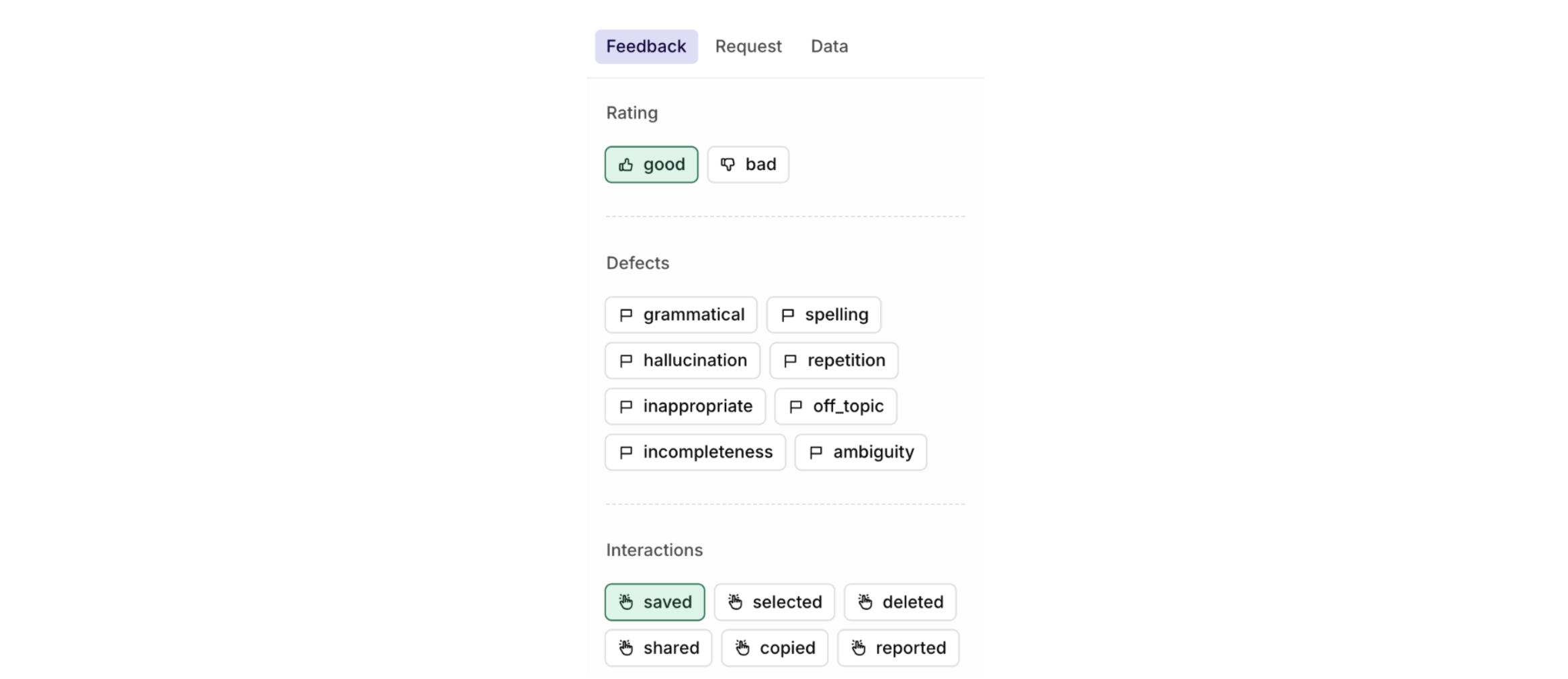
You can issue feedback in multiple ways:
- Through the orq.ai panel, see Feedback in Orq.
- Using the API, see Feedback via API.
By using corrections, feedbacks help you build human-curated Datasets. These datasets are used to train models with precise inputs and expected outputs, to learn more see Curated datasets.
Curated Datasets are used within experiments to verify performance and train models and make sure that they respond to expected user inputs.
Advantages of having a 'Human in the loop'
- Improved Quality and Trust: Human reviewers can help improve the quality and accuracy of LLM outputs, making them more reliable and trustworthy. This is particularly important in applications where errors or biases can have significant consequences.
- Adaptability and Customization: Issuing feedback allow LLMs to be customized for specific tasks or industries. Human input ensures the model aligns with domain-specific requirements and can handle nuances and complexities.
- Ethical Control: Feedback can prevent generating harmful, biased, or inappropriate content by providing human oversight and moderation. This is essential for maintaining ethical standards.
- Continuous Learning: Human reviewers can provide feedback to LLMs, helping them learn from their mistakes and improve over time. This iterative feedback loop contributes to the ongoing refinement of the model.
- Compliance and Regulation: Having a human in the loop can ensure that LLM outputs conform to legal and industry standards with strict guidelines in regulated industries or areas.
Updated 6 months ago