OpenAI - Prompt Management
This article guides you through integrating your SaaS with orq.ai and OpenAI using our Python SDK. By the end of the article, you'll know how to set up a prompt in orq.ai, perform prompt engineering, request a prompt variant using the SDK code generator, map the orq.ai response with OpenAI, send a payload to OpenAI, and report the response back to orq.ai for observability and monitoring.
This guide shows you how to integrate your products with OpenAI using the orq.ai Python SDK.
Step 1: Install the SDK
# orq.ai sdk
pip install orq-ai-sdk
# OpenAI
pip install openai
// orq.ai sdk
npm install @orq-ai/node --save
// OpenAI
npm install --save openai
Step 2: Enable models in the Model Garden
Orq.ai allows you to pick and enable the models of your choice and work with them. Enabling a model(s) is very easy; all you have to do is navigate to the Model Garden and toggle on the model of your choice.
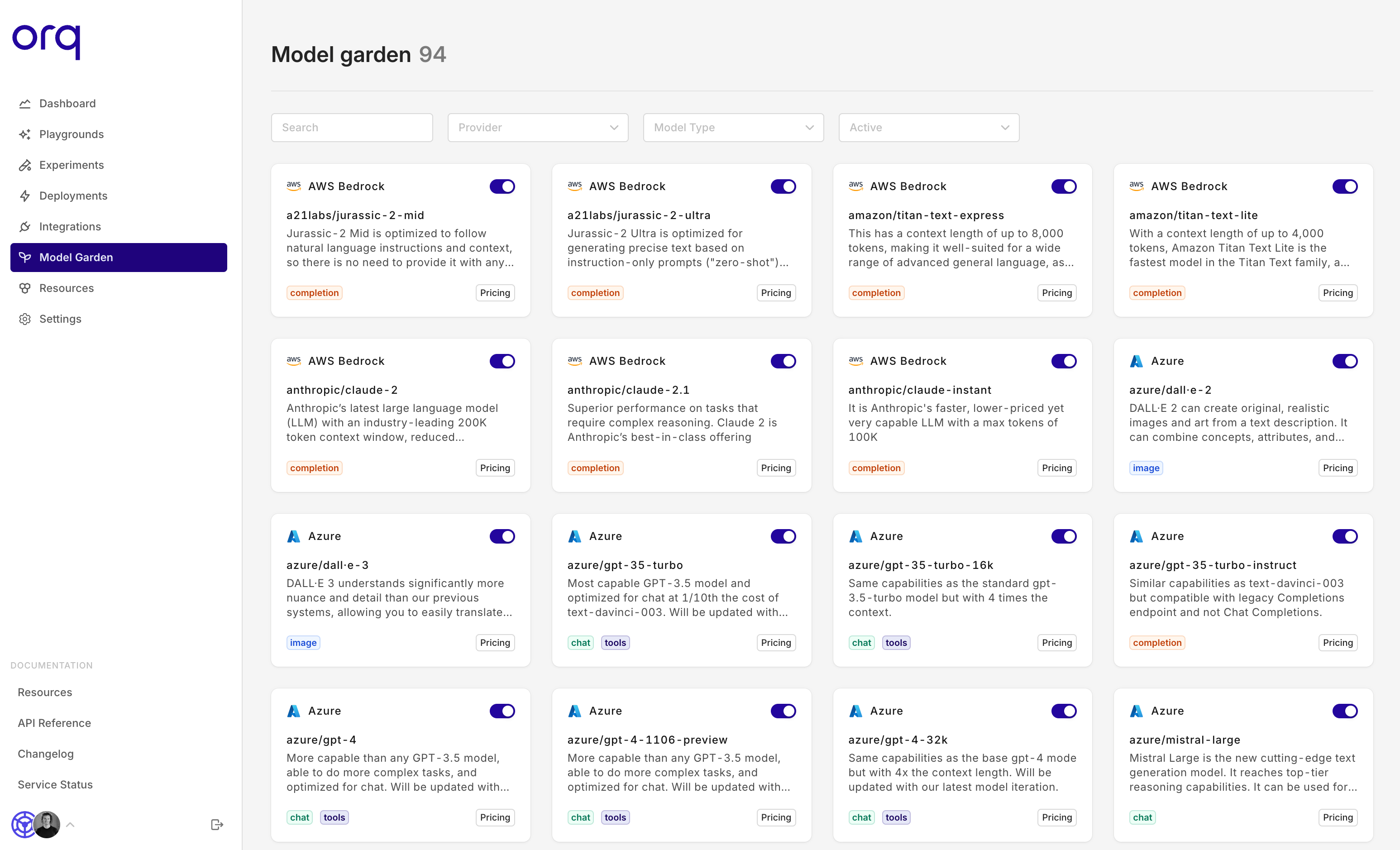
Step 3: Execute prompt
You can find your orq.ai API key in your workspace <https://my.orq.ai/><workspace-name>/settings/developers
from openai import OpenAI
from orq_ai_sdk import OrqAI
api_key = "ORQ_API_KEY"
# Initialize OrqAI client
client = OrqAI(
api_key=api_key,
environment="production"
)
# Getting the deployment config
deployment_config = client.deployments.get_config(
key="Deployment-with-OpenAI",
context={ "environments": [ "production" ], "country": [ "NLD", "BEL" ], "locale": [ "en" ], "user-segment": [ "b2c" ] },
inputs={ "customer_name": "John" },
metadata={"custom-field-name":"custom-metadata-value"}
)
config = deployment_config.to_dict()
# Send the payload to OpenAI
client = OpenAI(
api_key = "OPENAI_API_KEY",
)
chat_completion = client.chat.completions.create(
messages= config['messages'],
model=config['model'],
)
# Print the response
print(chat_completion.choices[0].message.content)
import { createClient } from '@orq-ai/node';
import OpenAI from 'openai';
//Initialize ORQ.AI client
const client = createClient({
apiKey: 'ORQ_API_KEY',
environment: 'production',
});
//Fetch Deployment Configuration
const deploymentConfig = await client.deployments.getConfig({
key: "deployment-with-OpenAI",
context: {
environments: [|"production"],
country: ["NLD", "BEL"],
locale: ['en']
},
metadata: {
"custom-field-name": "custom-metadata-value"
}
});
const openaiApiKey = 'OPENAPI_KEY';
// Initialize OpenAI
const openapi = new OpenAI({
apiKey: openaiApiKey,
});
// Request Chat Completion
const chatCompletion = await openapi.chat.completions.create({
messages: deploymentConfig.messages,
model: deploymentConfig.model,
});
// Print the response
console.log(chatCompletion.choices[0].message.content);
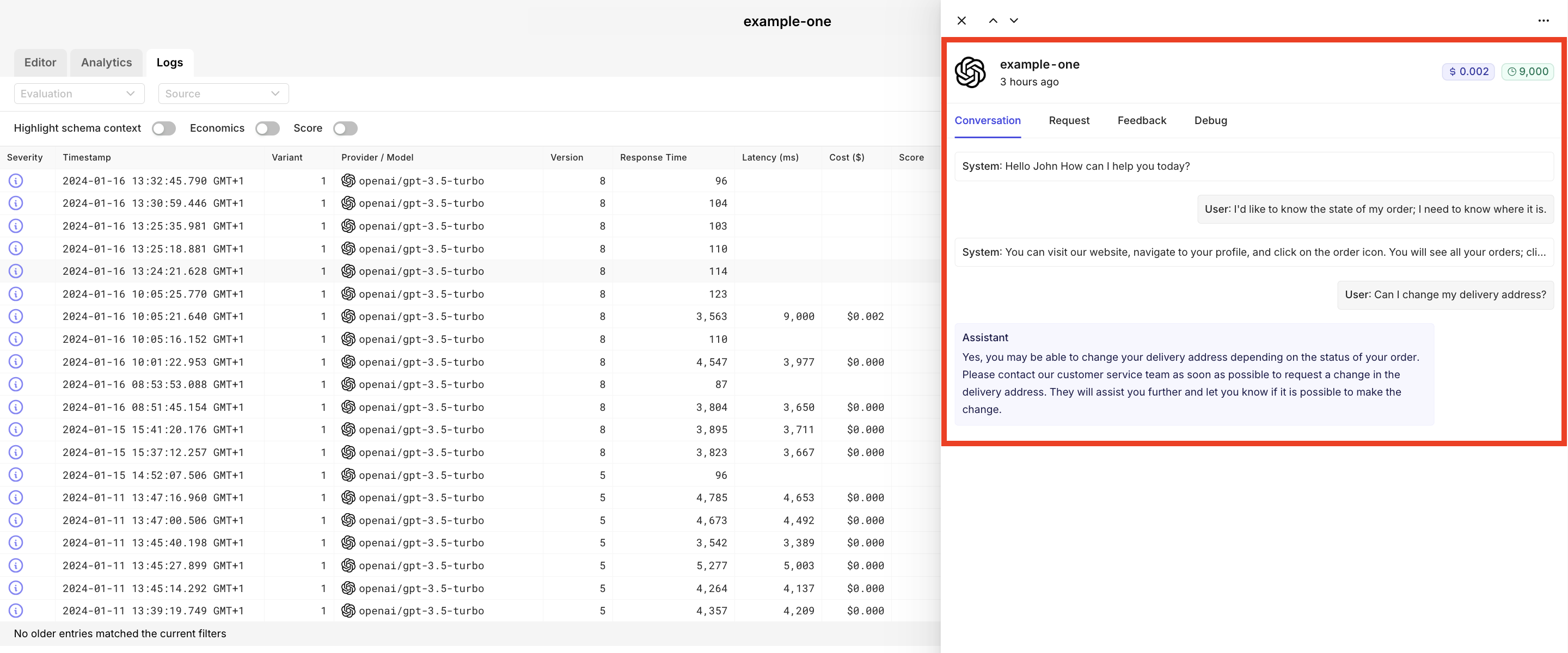
Step 4: Additional metrics to the request
After receiving your results from OpenAI, add metrics to the transaction using the add_metrics
method to complete the missing data for your Logging and Monitoring
# Additional informatiom
deployment_config.add_metrics(
chain_id="c4a75b53-62fa-401b-8e97-493f3d299316",
conversation_id="ee7b0c8c-eeb2-43cf-83e9-a4a49f8f13ea",
user_id="e3a202a6-461b-447c-abe2-018ba4d04cd0",
feedback={"score": 90},
metadata={
"custom": "custom_metadata",
"chain_id": "ad1231xsdaABw",
},
usage={
"prompt_tokens": 100,
"completion_tokens": 900,
"total_tokens": 1000,
},
performance={
"latency": 9000,
"time_to_first_token": 250,
}
)
deploymentConfig.addMetrics({
chain_id: "c4a75b53-62fa-401b-8e97-493f3d299316",
conversation_id: "ee7b0c8c-eeb2-43cf-83e9-a4a49f8f13ea",
user_id: "e3a202a6-461b-447c-abe2-018ba4d04cd0",
feedback: {
score: 100
},
metadata: {
custom: "custom_metadata",
chain_id: "ad1231xsdaABw"
},
usage: {
prompt_tokens: 100,
completion_tokens: 900,
total_tokens: 1000
},
performance: {
latency: 9000,
time_to_first_token: 250
}
})
Updated about 1 month ago